music.producer // ai.researcher
i produce music and do research in artificial intelligence
latest.music
contact: janne.spijkervet at gmail dot com
latest.projects
Anna’s Brains
First Dutch hip hop song written by AI
I appeared on VPRO’s Anna’s Brains on NPO3 to find out if we can make the first Dutch hip hop song written by AI for artist Daan Boom. The track was written by AI, and produced by me. You can learn more about the project here.
Klaas Kan Alles
Can we make a hit song with AI?
Klaas invited me on his show to embark on the challenge: can we write a hit song with AI? Without anyone knowing this track was written by AI or performed by Klaas, we submitted the track to The Netherlands’ most popular “music charts gatekeeper”, ‘Maak het of Kraak Het’. You can find out if our song made it on the charts here.
Tears on the Dancefloor:
A tearjerker written with Artificial Intelligence
In collaboration with SETUP.nl Utrecht and Pure Ellende, I designed the music and lyrics composition software that was used to create the first Dutch “smartlap” song. You can read more about the project at setup.nl/smartlap
tv // radio
film
publications
abstract
While supervised learning has enabled great advances in many areas of music, labeled music datasets remain especially hard, expensive and time-consuming to create. In this work, we introduce SimCLR to the music domain and contribute a large chain of audio data augmentations, to form a simple framework for self-supervised learning of raw waveforms of music: CLMR. This approach requires no manual labeling and no preprocessing of music to learn useful representations. We evaluate CLMR in the downstream task of music classification on the MagnaTagATune and Million Song datasets. A linear classifier fine-tuned on representations from a pre-trained CLMR model achieves an average precision of 35.4% on the MagnaTagATune dataset, superseding fully supervised models that currently achieve a score of 34.9%. Moreover, we show that CLMR’s representations are transferable using out-of-domain datasets, indicating that they capture important musical knowledge. Lastly, we show that self-supervised pre-training allows us to learn efficiently on smaller labeled datasets: we still achieve a score of 33.1% despite using only 259 labeled songs during fine-tuning. To foster reproducibility and future research on self-supervised learning in music, we publicly release the pre-trained models and the source code of all experiments of this paper on GitHub.
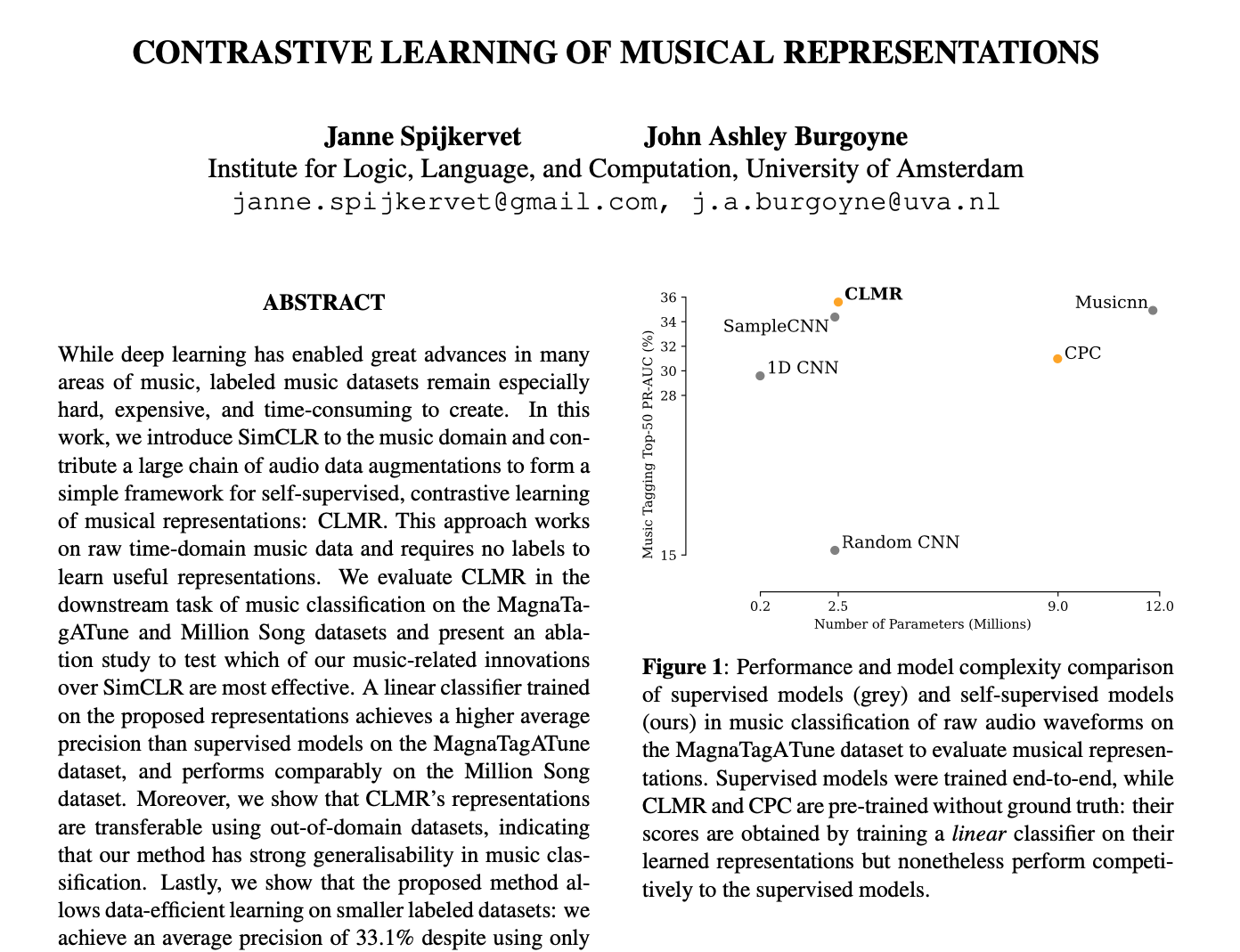
code
stories
scores
motivating.words
there is a vitality
a life force
a quickening that is translated through you into action
and because there is only one of you in all time
this expression is unique
but no artist is pleased
there is no satisfaction whatever at any time
only a queer divine dissatisfaction
a blessed unrest that keeps us marching
and makes us more alive
than the others
– Martha Graham